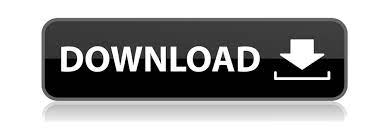
The model has not learned the meaning of words, but consider: While some of the sentences are grammatical, most do not make sense. His lordship pluck'd from this sentence then for prey, To stay him from the fatal of our country's bliss. O, no, no, no, no, no, no, no, no, no, no, no, no, no, no, no, no, no, no, no, no, it is no sin it should be dead,Īnd love and pale as any will to that word.īut how long have I heard the soul for this world,Īnd show his hands of life be proved to stand. The cause why then we are all resolved more sons. To watch the next way with his father with his face? Marry, and will, my lord, to weep in such a one were prettiest
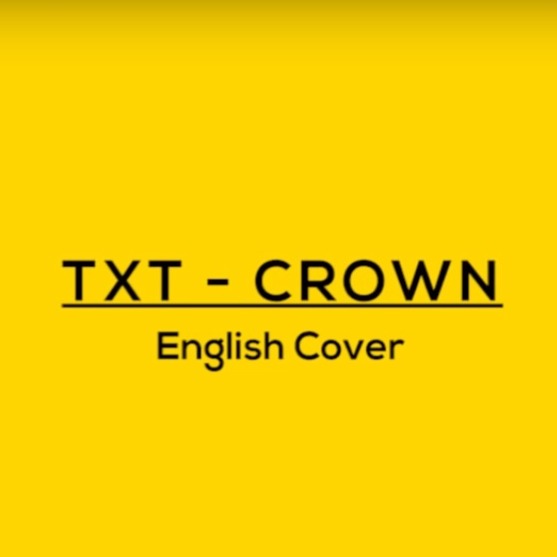
Which are so weak of care, by old care done Īnd the precipitation through the bleeding throne. Thus by All bids the man against the word, I had thought thou hadst a Roman for the oracle, The following is the sample output when the model in this tutorial trained for 30 epochs, and started with the prompt "Q": This tutorial includes runnable code implemented using tf.keras and eager execution.
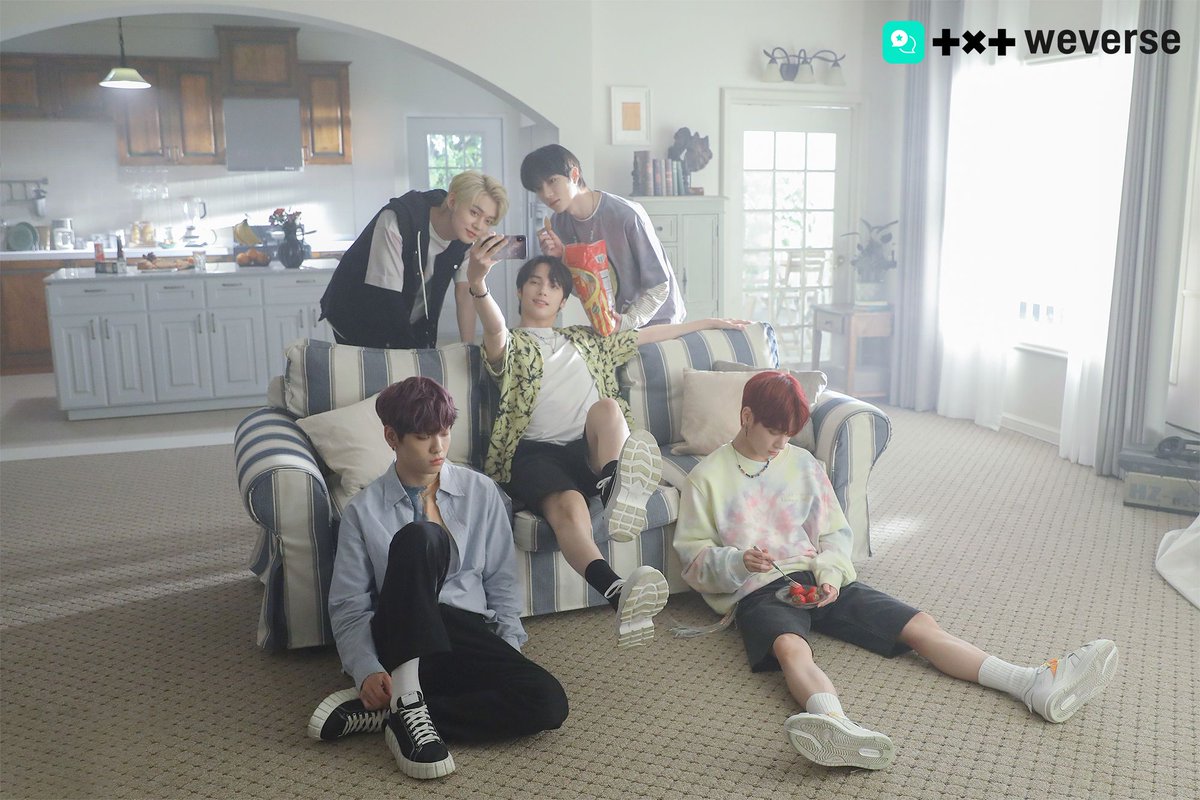
In Colab: Runtime > Change runtime type > Hardware accelerator > GPU. Note: Enable GPU acceleration to execute this notebook faster. Longer sequences of text can be generated by calling the model repeatedly. Given a sequence of characters from this data ("Shakespear"), train a model to predict the next character in the sequence ("e"). You will work with a dataset of Shakespeare's writing from Andrej Karpathy's The Unreasonable Effectiveness of Recurrent Neural Networks. This tutorial demonstrates how to generate text using a character-based RNN.
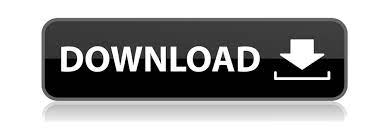